Center for Information Systems & Data Science
Research: Technology & Applications
The study of large-scale biomedical data sets, to better understand how living systems work, is known as health data science. A complex combination of genetic, environmental, and human variables influences many human diseases. By gathering, analyzing, and interpreting multidimensional data, health data science can give practical clinical information for clinicians and patients to better understand and avoid these complicated diseases.
Data-driven analysis of current health-care concerns has become possible thanks to the increased access to electronic health-record data. By creating revolutionary data science approaches, machine learning, and digital health modeling, data science helps us turn multidimensional biomedical data (such as multi-omics, imaging, wearable sensors, and electronic health record data) into practical health information.
Genomic research is one of the most important areas of health data science. A deeper understanding of the genome and the ability to use the genomic dataset are vital as the genomic revolution revolutionizes medical findings. Genomic data science is a subject that allows researchers to decode hidden functional information in DNA sequences using advanced computational and statistical approaches. Genomic medicine uses data science technologies to help researchers figure out how genetic differences affect human health and disease. Development of electronic health wearables in recent years also provides a wide range of data that combined with genomic and other health data sources will play a key major role in applications of data science in health and medical science in coming years.
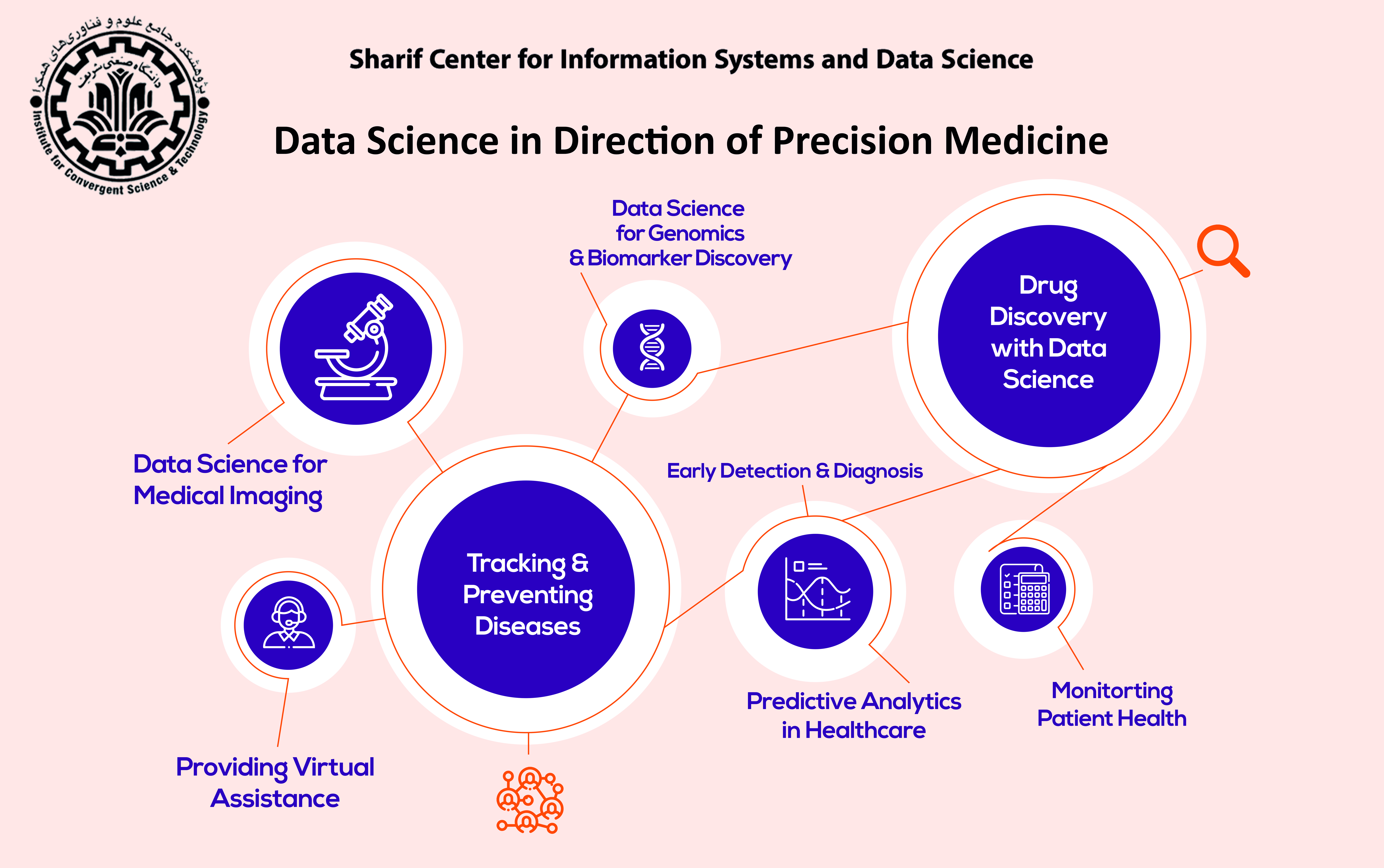
Other branches of this field include the use of artificial intelligence in structural biology. Structural biology is a branch of science that studies proteins and other biological molecules through their 3D structures. Measuring and interpreting biomolecular structures has traditionally been very difficult and expensive. Recently, it has been shown that become routine to predict and reason about structure at proteome scales with an unprecedented atomic-resolution with model-based-machine learning. This success will have a transformative effect on our ability to create effective drugs, understand engineer biology, and design new molecular materials and machines.
Computational biology is the science of using biological data to develop algorithms or models to understand biological systems and relationships. In the last few decades, with the expansion of the use of artificial intelligence in this field, researchers have the opportunity to develop analytical methods to interpret large amounts of biological information and use these algorithms to understand biological systems and relationships.
One of the achievements in this field is AlphaFold. AlphaFold is an artificial intelligence application developed by Google's DeepMind team that predicts protein structure. Proteins play a very important role in basically any important activity that takes place in the body of any living thing. Such as digestion of food, activation of muscles for contraction, movement of oxygen throughout the body, and attack of foreign viruses and bacteria. Proteins can perform a wide range of different functions, so they must be structurally capable of being transformed into complex 3D structures, therefore the number of different configurations that a protein may form based on its amino acid sequence is a very large number and theoretically, each protein can take on about 10^300 different structures. Therefore, estimating and determining the 3D structure of proteins was unattainable before the advent of AlphaFold, and AlphaFold revolutionized biology. Two years later, a newer version of the program called AlphaFold2 was developed in 2021, which greatly reduced the computational cost compared to the previous version. The main reason for this is that instead of learning each module separately in the model, it is based on the concept of Attention.
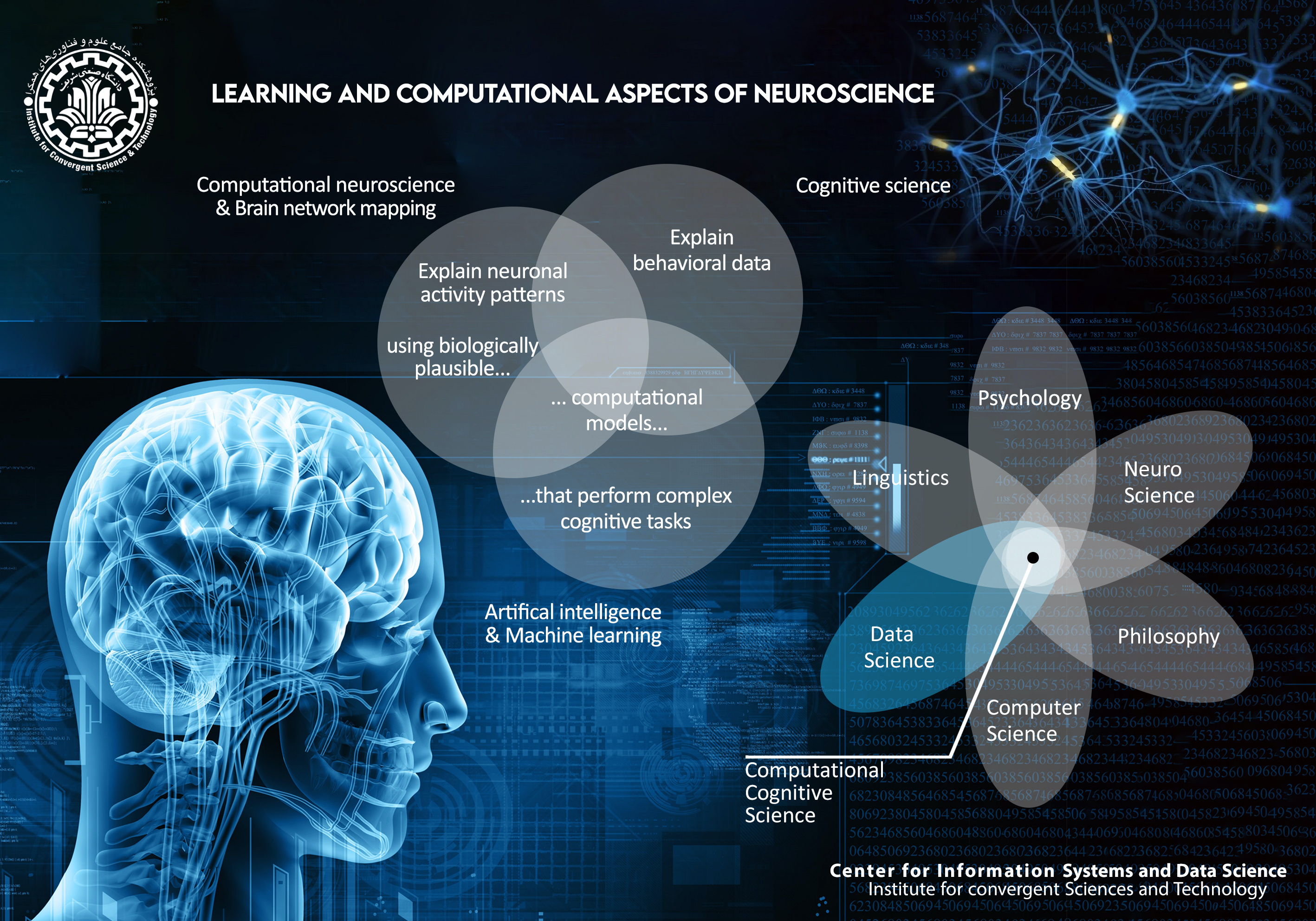

Data science for telecom operators to offer better services to clients
companies to obtain insightful information for making data-driven decisions faster and better than ever. The deeper the knowledge of customer preferences and needs, the more profit can be gained by the telecom operators by having better understandings of their customers. Such data-driven mechanisms include:
- Customer segmentation
- Customer churn prevention
- Customer lifetime value prediction
- Recommendation engines
- Customer sentiment analysis
- Real-time analytics
- Price optimization
Data science for telecom operators to improve the networking
Taking advantage of data science techniques, such as real-time data analytics, the telecom service providers can accurately discover highly congested areas, and adopt proper network traffic optimization methods intelligently. Data science in telecom can also facilitate detecting anomalies and ensure that the network systems work securely, reliably, and efficiently.
b. Fraud Detection:
Based on industry estimates, telcos annually lose approximately 2.8% of their revenues to leakage and fraud, costing the industry approximately US $40 billion every year. Telecom industry-being the one attracting a significant number of users per day-is vulnerable to “fraudulent activities.” Some of the widespread cases of fraud in the context of telecom industry are illegal access or authorization, theft or fake profiles, cloning, behavioral fraud, etc. Fraudulent activities directly affect the relationship established between the operator and the clients. Therefore, utilizing intelligent data-driven methods, offered by data science, for fraud detection systems are critically important for telecom service providers.
Edge AI
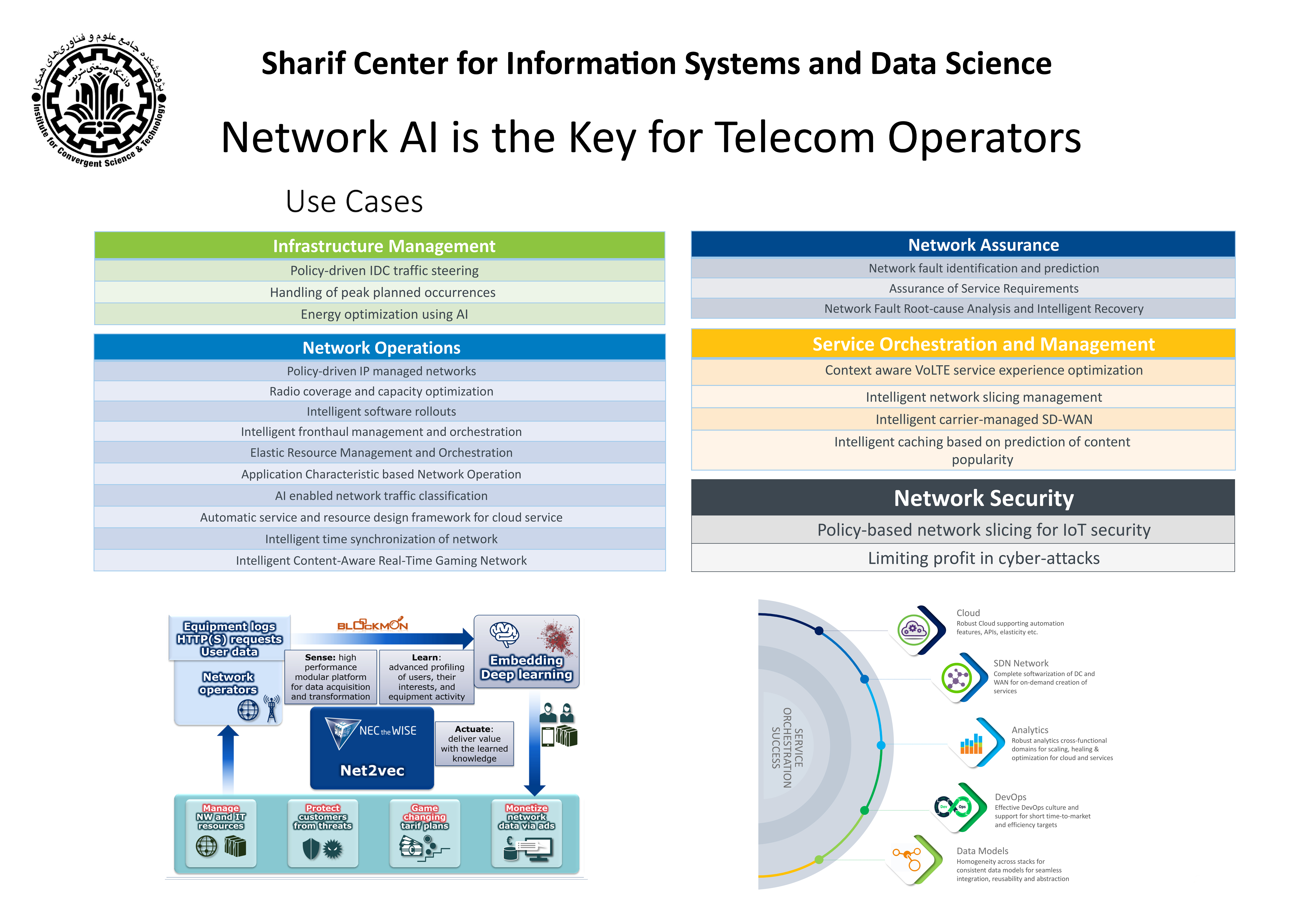
The occurrence of traffic, accident, or vehicle breakdown, for example, can be identified or predicted using transportation data science, and effective responses suggested that new approaches solve traffic problems, improve transportation safety, system efficiency, and quality of life in our society.
The main areas of transportation include roadways, railways, waterways, and airlines.

Road safety management
Road traffic management
Rail traffic management
Air traffic management
Ship monitoring and route optimization
- When should the body be cleaned to save fuel?
- When should ship equipment be replaced?
- Which is the best route in terms of climate, safety, and sustainable fuel?
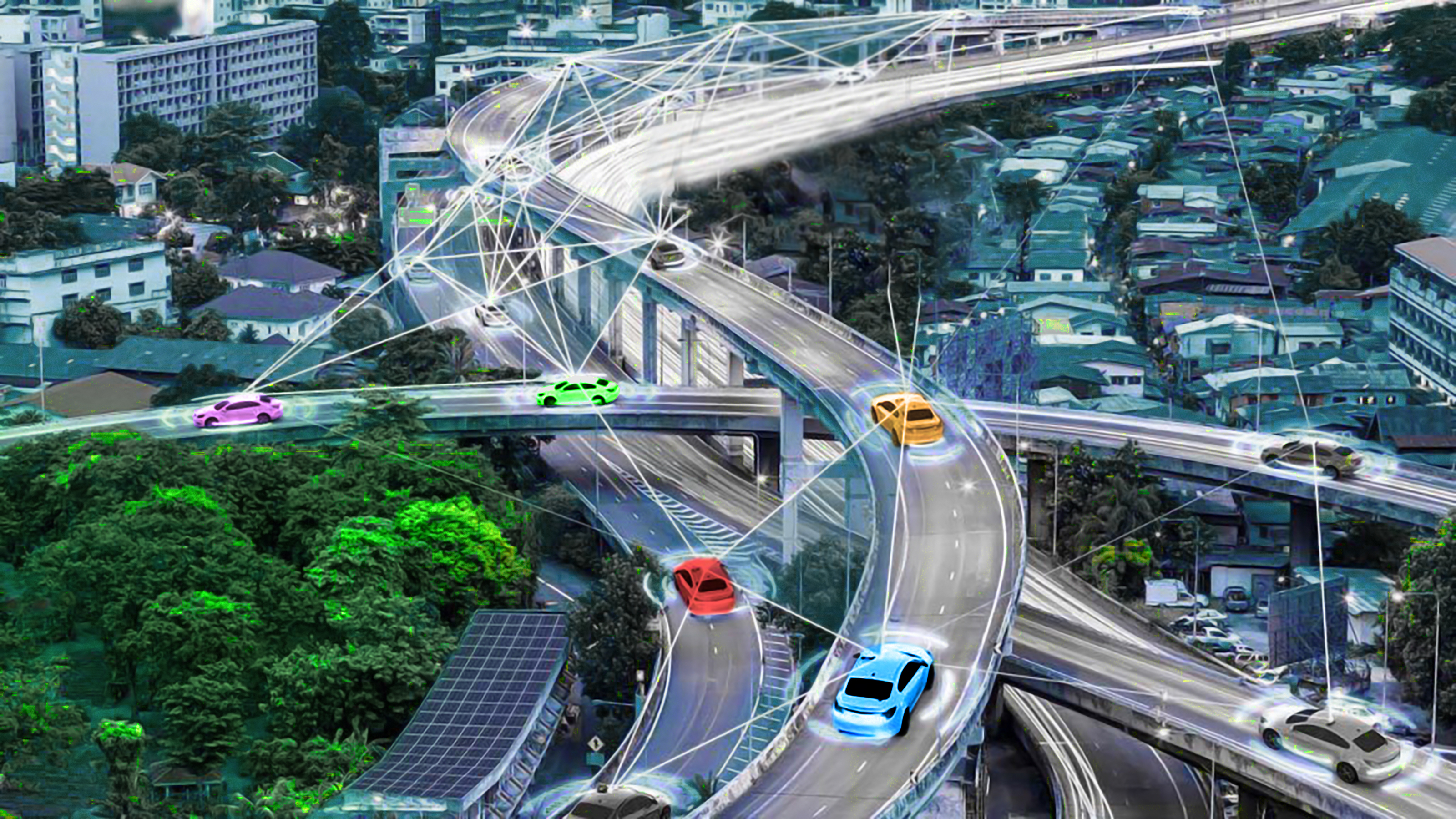
Digital media is very broad and its nature and data sources are very diverse (for example, text, audio, images and videos on news websites and social media platforms). Media-related data is inherently time-dependent, and often there is not enough ground truth data for many of the questions of interest (e.g., news credibility of the level of articles or claims). The diversity of media data raises important questions about the generalizability of scientific tools across platforms, languages, and data formats, and the interactive aspect of media raises profound questions about causality. Research challenges in digital media have been explored from a variety of angles, including natural language processing, network science, machine learning, statistics, computer science, social science, and political science. One of the main challenges of data science is the processing of this type of data. This processing includes steps such as storing, managing, analyzing and integrating data in an optimal way. Another challenge of data analysis is the simultaneous management of structured and unstructured data. Unstructured data is usually a combination of different types of data such as text, images and video that are generated from different sources. It is not easy to use normal methods in storing this data and their processing requires new methods.
In this group, we hope to define and address real-world challenges with the help of researchers.
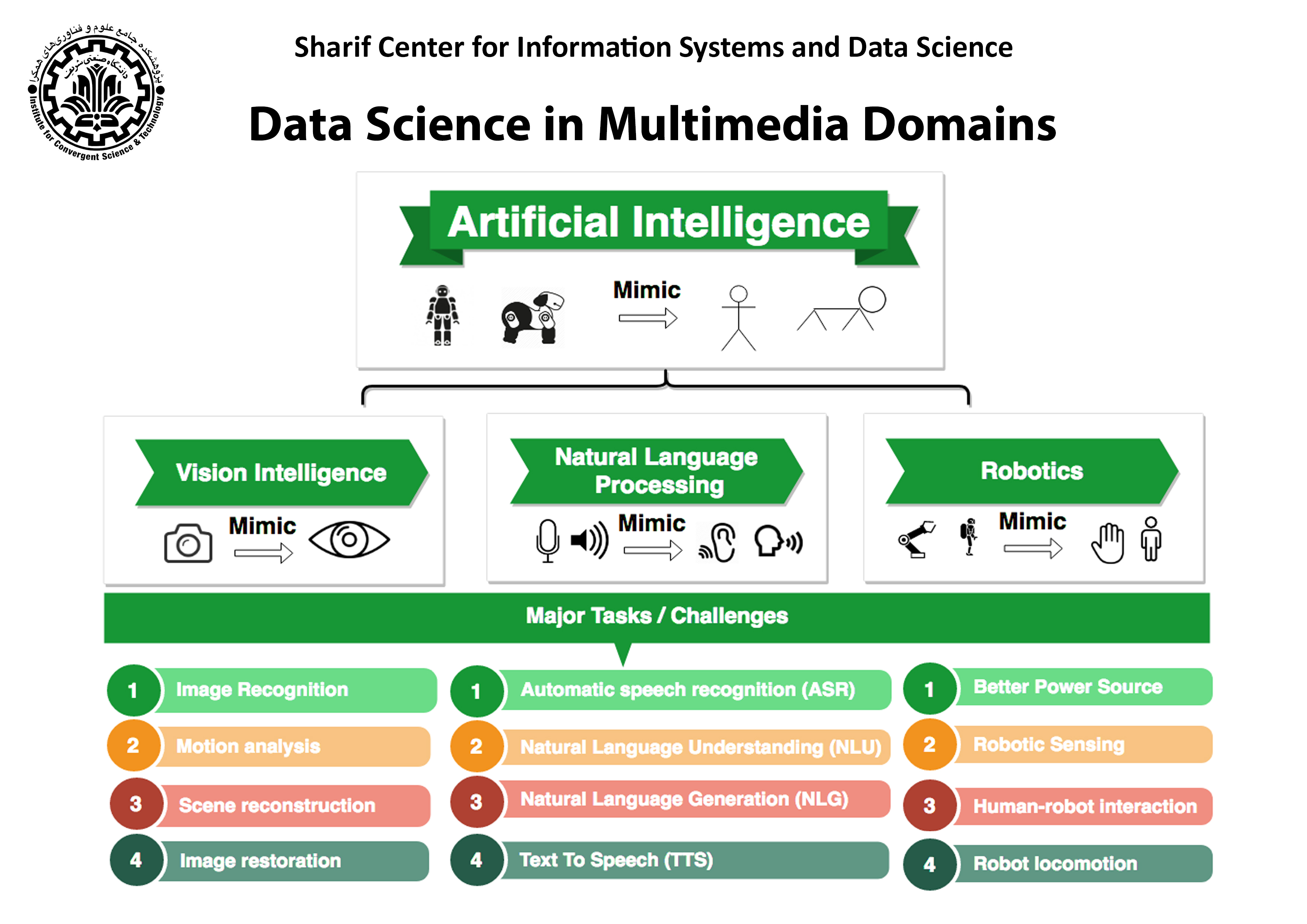
The application of data science techniques to financial challenges is known as financial data science. Computer science, mathematics, statistics, information visualization, graphic design, complex systems analysis, communications, and business knowledge are all used in financial data science. Forecasting models, clustering, resolving data controversy, visualization, and handling dimensionality are some of the most prevalent information extraction approaches that provide robust possibilities for interpreting financial data and solving related challenges.
The banking industry is one of the most profitable industries in the world. Banks have long endeavored to predict market developments in order to make the best investment and obtain competitive advantage. In such scenarios, data analysis reveals the most effective method for making these decisions. Banks and other financial institutions have access to our data and market data, ranging from market metrics to transaction data and client profiles, allowing them to play an essential role in this market. However, one of the most difficult issues in this field is figuring out how to make the most of the available unstructured data. This is where financial data scientists come into play. They can collect, extract, and analyse data to offer valuable information. A financial data scientist's responsibilities can range from fraud detection to developing individualized customer care solutions.
A new discipline called social data science integrates social sciences and data science. Big data analysis is linked to social science theory and analysis in this subject. The problem in this area is that although we can explain social phenomena, these justifications occur years after they actually occur.
To put it another way, we have yet to be able to "predict" social processes in a proper time. In this industry, data science can also be beneficial.
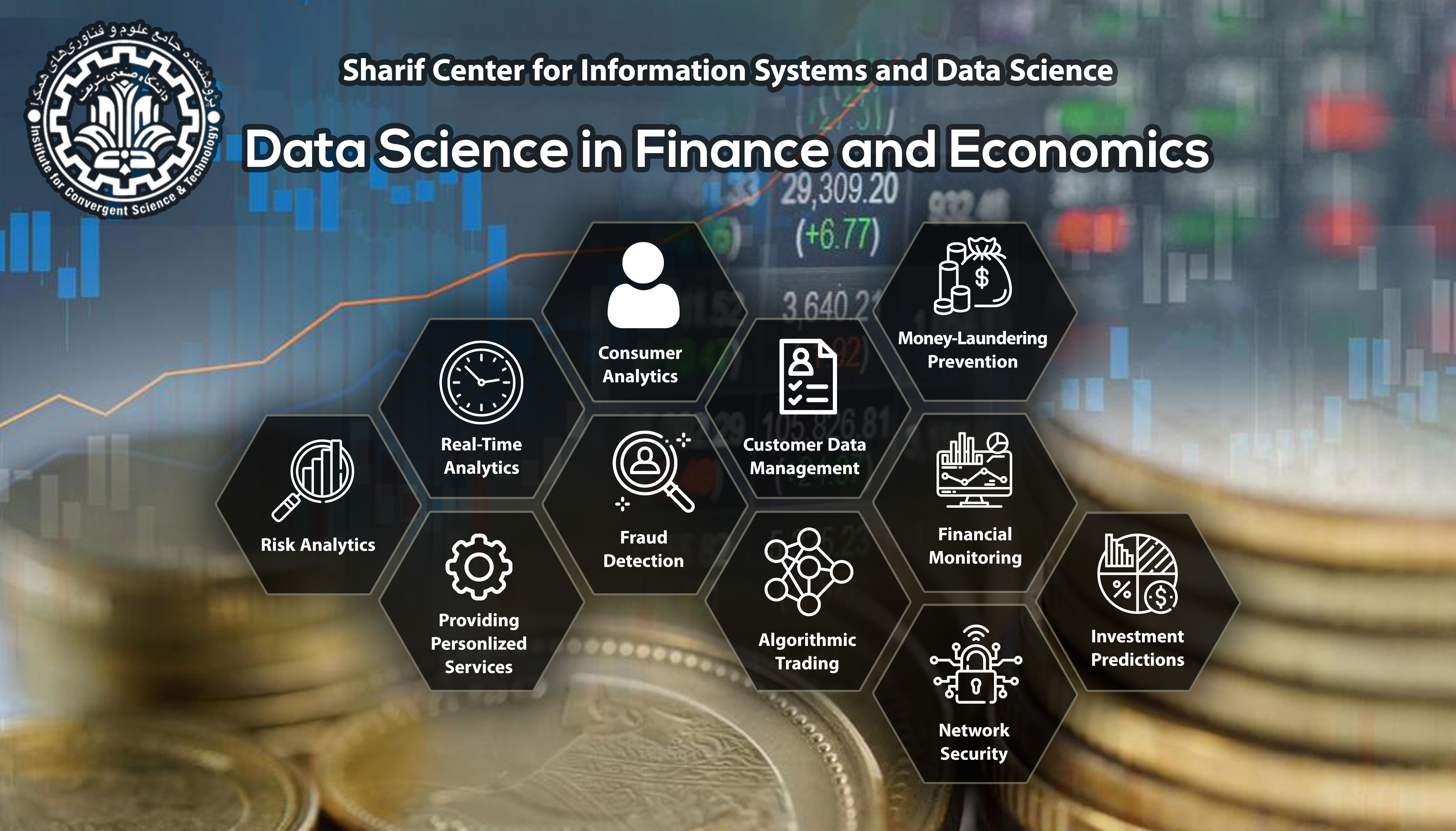
Using data science-based engineering methods, we can swiftly collect and analyze vast amounts of structured and unstructured data in order to uncover hidden patterns, new correlations, trends, customer insights, and other crucial business-related information. Moreover, new means of improving exploration and production can be identified. We, at Sharif Data Science Center can extract important insights from such large and valuable data.
Oil and gas companies face challenges such as inflexibility and unpredictability. Data science solutions may help oil and gas managers solve problems and achieve more efficient outcomes by bringing agility, clarity, and usability to the table. In order to make better and more informed decisions, one must extract insights from massive amounts of data. Using advanced analytics and artificial intelligence, oil and gas companies may find trends and predict occurrences throughout operations, allowing them to respond quickly to disruptions and boost efficiency.
For example, by evaluating data from transmission line and refinery safety inspections, data science can lead to the creation and development of algorithms and analytical forecasts to identify the safety status of lines. In addition, it will be possible to recognize dangerous trends and locations intelligently, as well as detecting security and safety issues quickly to deliver timely warnings.
In the energy industry, data science may also play a key role in automating and improving resource management and consumption. Some of the uses of data science in this industry include providing accurate projections of energy consumption trends and recommending appropriate options to boost productivity and resource efficiency. By overcoming human limits in analysis, forecasting, and decision making, data science and artificial intelligence assist these businesses in performing extraction, processing, and production processes with maximum speed, least error, and maximum efficiency.
At the management level, artificial intelligence can deliver services such as equipment error detection and prediction, security and safety, dependability analysis, demand and price forecasting, to name a few.

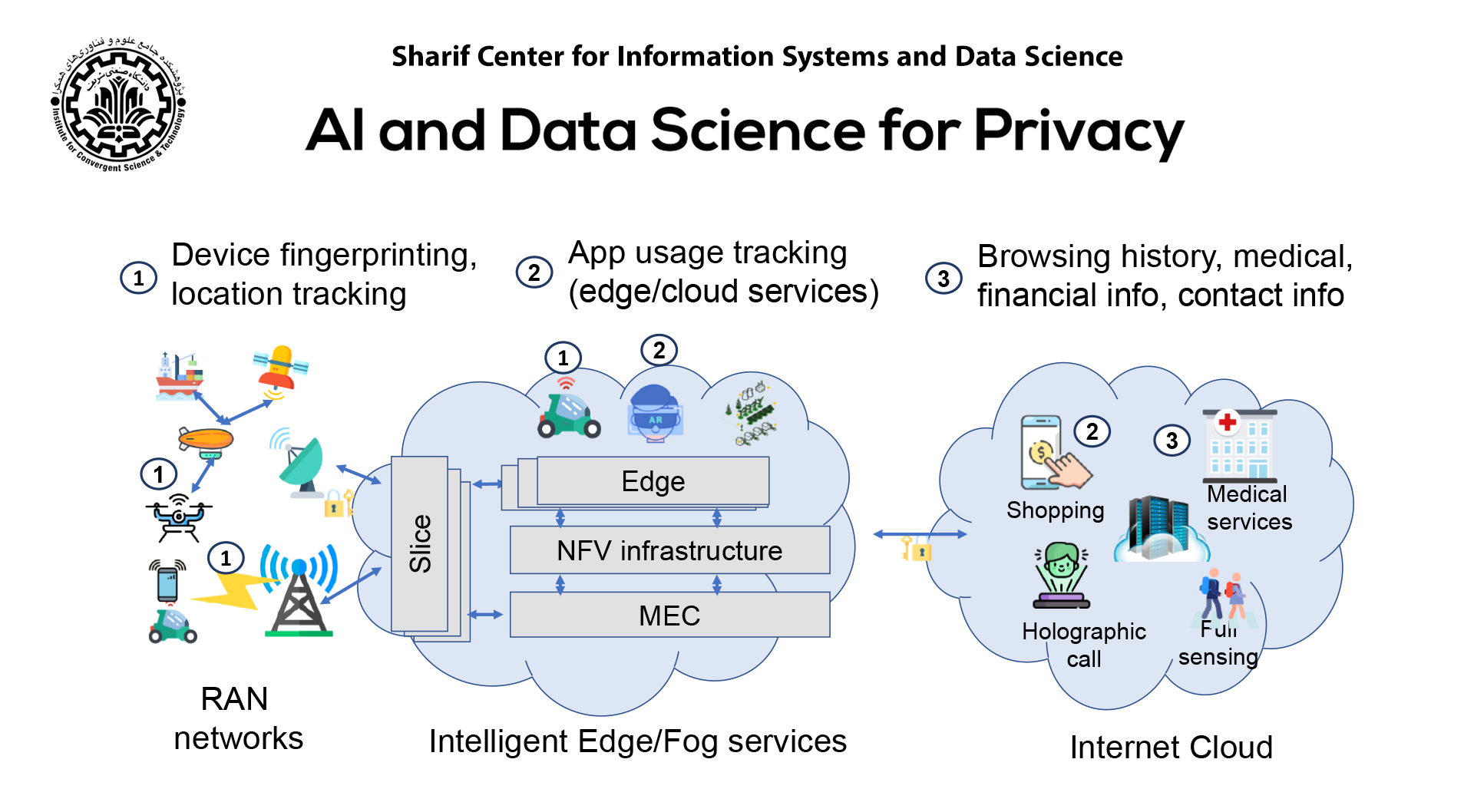
Cryptography and security algorithms were conventionally developed to focus on specific solutions for the applications of banking or communications. Recently, as a result of the amazing advances made in the areas of data science and computer science, a wide range of applications and systems require ubiquitous security and privacy guarantees. Examples include, but not limited to, connected cars, digital healthcare services, smart factories and smart buildings.
We need security and privacy solutions that work well in practice. To this end, it is required to draw insights from empirical and behavioral data. Therefore, security and privacy guarantee mechanisms for real-world applications that are capable of keeping pace with the continuing growth of IT infrastructures are needed to provide empirical methods for dealing with heterogeneous datasets on hand.
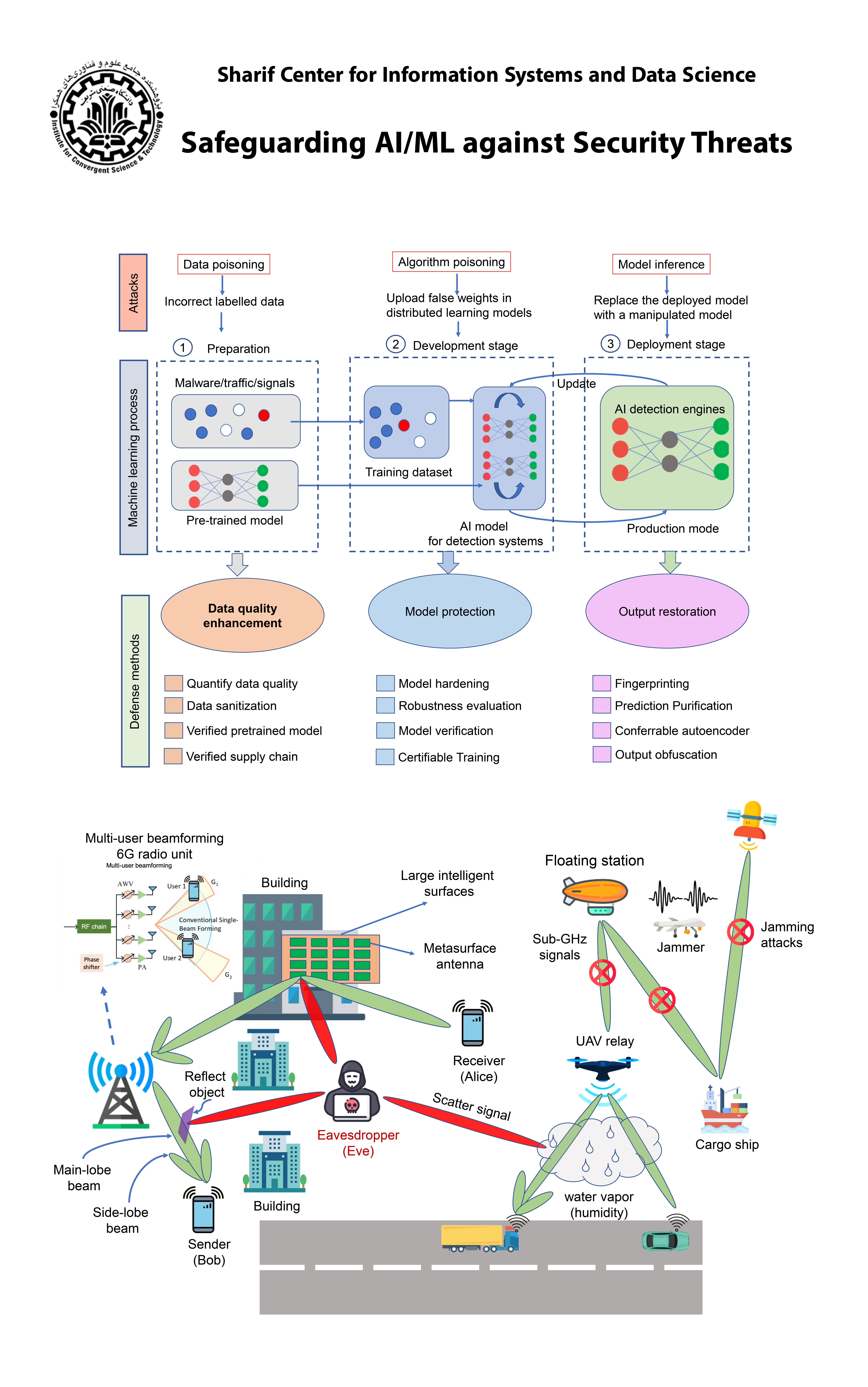
Physics and astronomy aren't the only physical sciences that are relying more and more on big data analysis. In recent years, thorough, diligent, and high-tech observation has fueled advances in geophysics and climate science/meteorology. Seismologists began mapping the Earth's internal structure in 3D models in the 1980s. Other data-driven advances were made possible by GPS data that was able to capture the ultra-slow motion of tectonic plates that had been known to occur over millions of years but also demonstrated how the plates were internally deforming over years to decades. Seismologists were the first scientists to publicly share their data with the rest of the world!
The capacity to investigate matter at the nanoscale, as well as the greater use of computer simulations to forecast physical and chemical characteristics of materials, has also resulted in innovative methodologies in chemistry and materials science and engineering.
Moreover, the rapid evolution of Internet-connected gadgets that provide observations and data exchange from the physical world has been forced by the rapid rise of software, hardware, and communication devices and technologies. The Internet of Things (IoT) is a network of physical objects embedded with sensors, software, and other technologies to connect and exchange data with other devices and systems through the internet. These devices range in complexity from common household items to sophisticated industrial instruments. In simple words, the Internet of Things (IoT) is a collection of internet-connected devices that exchange data in order to improve their performance; these are automatic processes that do not require human interaction or input. Analysis of such amount of physical data is also at the forefront of data science objectives in the coming years.
Space-Air-Ground Integrated Networks and Aeronautical Ad-hoc Networking
With the growth of transcontinental air traffic, the newly-emerged concept of aeronautical ad hoc networking, which relies upon commercial passenger airplanes, is potentially able to improve satellite-based maritime communications through air-to-ground and air-to-air links.
Due to the fact that more than 70 percent of the Earth surface is covered by oceans, the increasing activities scattered across the ocean have made great demand for maritime communications. Nowadays, shipping mainly relies on satellites for seamless coverage. Nevertheless, due to the wide coverage area of a satellite, the allocated bandwidth per user is actually limited. In addition, there is an increasing number of intercontinental passenger airplanes above the ocean, resulting in an ever-rising demand for in-flight Internet connectivity. Similar to ships, airplanes also face the same satellite connection limitations. Therefore, the concept of aeronautical ad-hoc networking is proposed to form a self-configured wireless network via air to-air communication links. In other words, it is a nature inspiration to conceive the combination of satellites and airplanes to form a space-air-ground integrated network for supporting future maritime communications. Notably, the design and optimization of space-airground integrated networks face numerous challenges! A fundamental one, for example, is to design an efficient routing protocol for constructing possible data routing at any given time with the aim of being compatible with the highly-dynamic network topology. In such applications, the utilization of ideas, algorithms, and tools related to data science are highly required to come up with novel data-driven solutions for the future space-air-ground integrated networks.